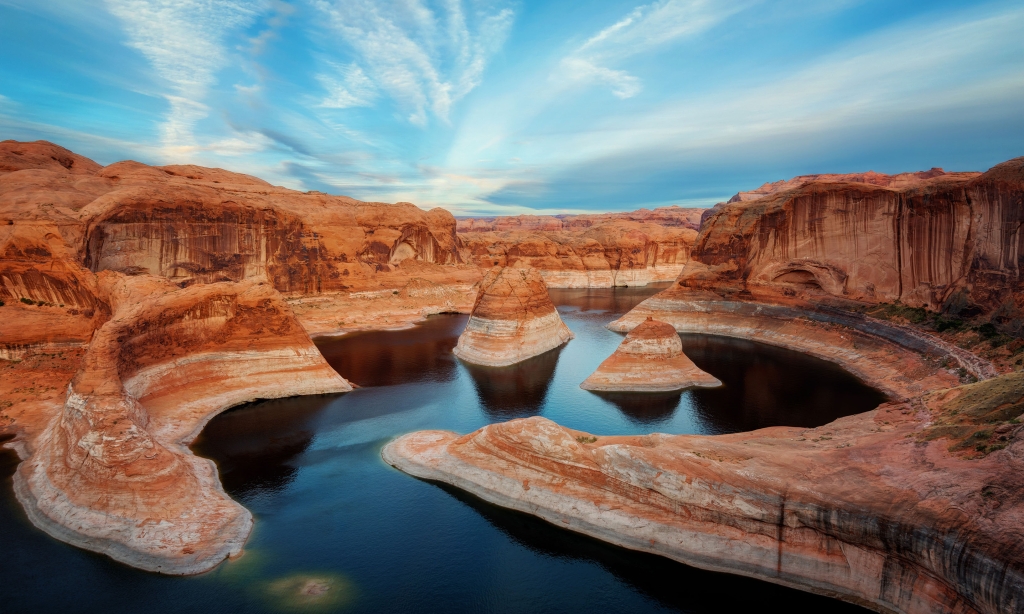
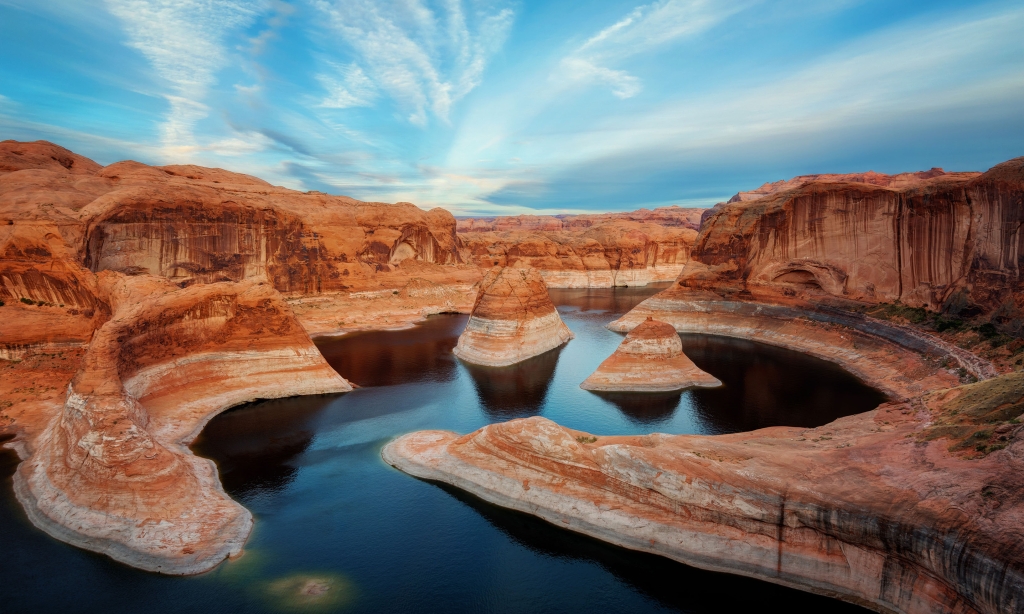
Insights from
Written by
You’re taking a red-eye to Europe and wake up bleary-eyed to find that you are landing in Heathrow. Any excitement you have about closing in on your final destination is quickly dampened by the thought of what awaits you on the ground: the dreaded airport transfer.
Some 80 million passengers travel through London’s Heathrow Airport every year, and 30 million of them are there only to pass through to another city — often trapped in long lines at customs and immigration and scrambling to get to the next flight before it leaves.
“Once passengers land, there is a very little data that is connected on them,” says Yael Grushka-Cockayne, Altec Styslinger Foundation Bicentennial Professor of Business Administration and senior associate dean for professional degree programs at Darden. “Traditionally, the airport doesn’t really know where people are, and it doesn’t really anticipate where they are going to be.” Several years ago, Grushka-Cockayne was hired by Eurocontrol, an agency supporting European aviation, to try to remedy the situation. With colleagues from University College London, she developed a model to better predict the flow of passengers through Heathrow’s transfer terminals, publishing the results of their research in two recent papers.
“I always like working with projects that are more tangible,” says Grushka-Cockayne, who has consulted with Eurocontrol ever since her dissertation at London School of Economics more than a decade ago. “In this case, we had the opportunity to demonstrate the benefits of our research, but we weren’t sure how far they were willing to take it.”
Grushka-Cockayne specializes in decision analysis, using data and computer modeling to make predictions that can optimize decision-making. In the case of Heathrow, she and her fellow researchers started by gathering as much data as they could. Though, like many airports, Heathrow centralized operations in an Airport Operations Center, the information collected there was highly fragmented. “Everybody was sitting right next to each other, but there was no sharing of data,” Grushka-Cockayne says. One database had information about the number of planes arriving, while another had information on where passengers were transferring. “We worked for ages to unify the data sets and come up with a way to compare variables.”
Previously, the airport created predictions based on past information that could be days or weeks old — often of little use in making predictions in the moment. Grushka-Cockayne and her colleagues took the information they had collected and created a machine-learning algorithm that could be trained to make accurate predictions in real time. “We wanted to make something that at 10 p.m. could predict what we planned to see at 11 p.m.,” she says, “as opposed to something that came from last week’s information.”
Knowing when an airplane would arrive and how many passengers were on it, however, wasn’t enough to accurately predict how many passengers would arrive at a checkpoint 20 minutes later. While business travelers might make a beeline for immigration, families might take significantly longer to amble through the transfer corridors. To take that into account, the researchers created a distribution of arrival times based on the actual population on the plane, based on probability rather than an absolute number.
Once they had that measure, the next step was to look at where the passengers were going based on their itinerary information, in order to give better estimates to departing airlines. “If a flight knows they have 12 passengers who are going to be 20 minutes late versus two passengers that are going to be an hour late, that could determine which of their flights might be delayed or forge ahead to leave on time,” Grushka-Cockayne says. Additionally, the researchers were able to give information to the airport operations about staffing levels, advising them on when, due to increased passenger traffic, they might add additional staffing to meet the demand.
In the end, the model ended up using a relatively simple machine-learning algorithm, which proved remarkably accurate in predicting actual counts of passenger flow. After the team presented its evidence, Heathrow implemented the system as a pilot in 2016, generating forecasts on a rolling basis with updates every 15 minutes and real-time predictions of late passengers for each flight — producing much more accurate predictions than its previous system.
The researchers estimate that the model reduces costs from overstaffing and dissatisfied customers anywhere between 20 and 54 percent. “We’re talking for sure in the millions of dollars in terms of savings,” Grushka-Cockayne says.
The airport was so pleased with the pilot program, it implemented the system permanently. The successful implementation has changed the mindset of airport management, which is now considering applying machine learning to optimize other aspects of operations. “Machine learning was no longer this buzzword used by outsiders, but they felt it was relevant to them,” says Grushka-Cockayne. Since then, she says, other hubs such as Los Angeles International Airport have approached her and the team about implementing a similar system.
The implications, however, go beyond airports, to be relevant to hospitals and other institutions that need to efficiently manage customer flow. “There are all kinds of industries in which you don’t want to overstaff because you will lose money, but you also don’t want to understaff because it will harm customer service,” says Grushka-Cockayne. “Anywhere that you need to move a lot of people — and increase your ability to predict their behavior — could benefit from this approach.”
In other words, while a system such as this one can’t completely take away the hassle of changing planes, it can at least make it a much less unpleasant experience — making arrival at that final destination all the more sweet.
Yael Grushka-Cockayne co-authored “London Heathrow Airport Uses Real-Time Analytics for Improving Operations” and “Forecasting Airport Transfer Passenger Flow Using Real-Time Data and Machine Learning” with Xiaojia Guo and Bert De Reyck , both of University College London School of Management.
Grushka-Cockayne’s research and teaching activities focus on decision analysis, forecasting, project management and behavioral decision-making.
As an expert in the area of project management, she has served as a consultant to international firms in the aerospace and transportation industries. She is the secretary/treasurer of INFORMS Decision Analysis Society, a U.Va. Excellence in Diversity fellow and member the Project Management Institute.
B.Sc., Ben-Gurion University; M.Sc., London School of Economics; M.Res., Ph.D., London Business School
Heathrow Airport Operations: Data Connections to Improve Flight Connections
Share